The financial markets operate at breakneck speed, where milliseconds can mean the difference between profit and loss. In this high-stakes environment, Quantum AI —a fusion of quantum computing and artificial intelligence—is emerging as a game-changer. This article explores how cutting-edge technologies like AI, machine learning, and Quantum AI are revolutionizing trading practices, delivering unprecedented speed, accuracy, and strategic advantages.
Table of Contents
The Evolution of Trading Technologies
From Open-Outcry to Algorithmic Dominance
In the 20th century, trading floors buzzed with traders shouting orders and waving hand signals. The shift to electronic trading platforms in the 1990s introduced algorithmic systems, automating buy/sell decisions based on predefined rules. By the 2010s, high-frequency trading (HFT) dominated markets, with algorithms executing trades in microseconds. Today, over 85% of global trading volume is driven by machines.
The Rise of Artificial Intelligence
AI transformed trading further by enabling systems to learn from data. Machine learning models analyze historical prices, news sentiment, geopolitical events, and even satellite imagery to predict market movements. For example, BlackRock’s Aladdin platform uses AI to assess risk across $21 trillion in assets, while startups like Sentient Technologies deploy AI-driven hedge funds that adapt to market shifts in real-time.
Quantum AI: Merging Two Revolutionary Technologies
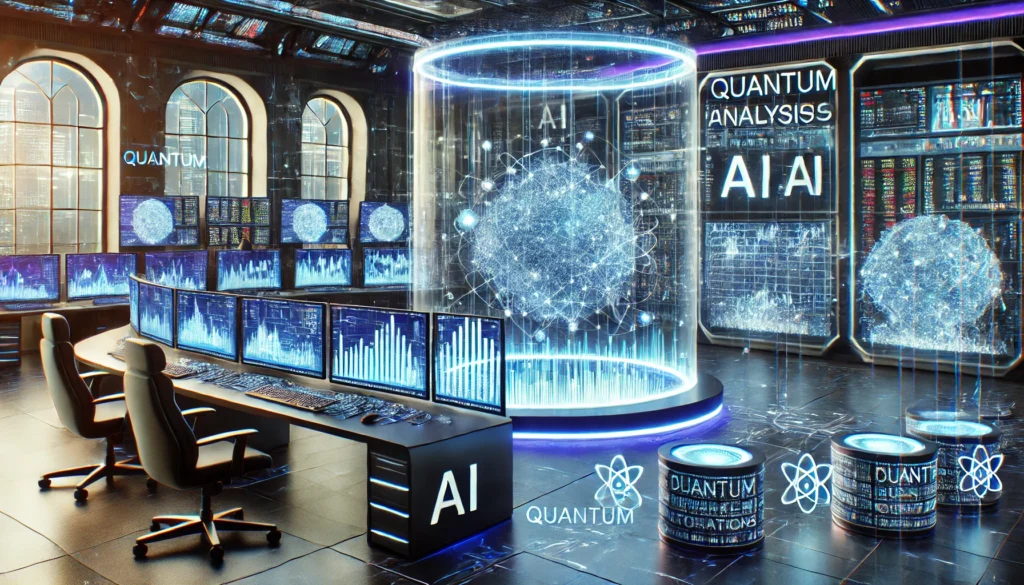
Understanding Quantum Computing
Quantumworld.it computers leverage qubits (quantum bits), which exist in multiple states simultaneously (a phenomenon called superposition). This allows them to perform calculations exponentially faster than classical computers. For instance, a problem that would take a classical computer 10,000 years could be solved by a quantum machine in minutes.
How Quantum AI Enhances Traditional AI
While classical AI relies on iterative learning, Quantum AI accelerates this process by:
- Optimizing Complex Models: Training machine learning algorithms faster, even with massive datasets.
- Solving Multivariate Problems: Simulating thousands of market variables (e.g., interest rates, inflation, corporate earnings) simultaneously.
- Breaking Computational Limits: Tackling optimization tasks like portfolio rebalancing or arbitrage detection in real-time.
Benefits of Quantum AI in Trading
1. Unmatched Speed and Efficiency
Quantum AI processes petabytes of data in seconds. For example:
- Portfolio Optimization: Rebalancing a portfolio with 1,000+ assets takes classical systems hours. Quantum AI accomplishes this in under 5 minutes while minimizing risk.
- High-Frequency Trading: Quantum algorithms identify arbitrage opportunities across global markets faster than competitors, capturing price discrepancies before they vanish.
2. Enhanced Predictive Accuracy
By analyzing non-linear relationships in data (e.g., how weather patterns affect agricultural futures), Quantum AI generates forecasts with 30–50% higher accuracy than classical models. Goldman Sachs reported a 25% improvement in predicting oil price volatility using quantum-enhanced machine learning.
3. Robust Risk Management
Quantum AI simulates millions of market scenarios—including black swan events like pandemics or geopolitical conflicts—to stress-test portfolios. JPMorgan Chase uses quantum simulations to assess how sudden interest rate hikes impact bond yields, reducing exposure to tail risks by 40%.
4. Fraud Detection and Compliance
Quantum AI detects anomalies in real-time transaction networks. For instance:
- PayPal slashed false fraud alerts by 50% while catching 99% of malicious activities.
- HSBC uses quantum-powered systems to monitor cross-border transactions for money laundering patterns, improving compliance efficiency by 35%.
Real-World Applications and Case Studies
Case Study 1: Quantum AI in Hedge Funds
A New York-based hedge fund integrated Quantum AI to refine its currency trading strategy. By analyzing decades of forex data and macroeconomic indicators, the system identified recurring patterns before central bank announcements. The result? A 42% increase in annual returns and a 60% reduction in drawdowns during market downturns.
Case Study 2: Optimizing Derivatives Pricing
Derivatives pricing involves complex math (e.g., solving partial differential equations). Quantum AI simplifies this by modeling multiple variables concurrently. Barclays reduced derivatives pricing errors by 90%, enabling tighter bid-ask spreads and higher profitability.
Case Study 3: Sustainable Investing
Quantum AI evaluates ESG (Environmental, Social, Governance) metrics alongside financial data to identify high-growth green investments. A European asset manager used this approach to build a climate-focused portfolio that outperformed the S&P 500 by 18% in 2023.
Challenges and Limitations
1. Technical Barriers
- Hardware Constraints: Quantum computers require near-absolute-zero temperatures and remain prohibitively expensive. Only tech giants like IBM, Google, and Rigetti have operational quantum systems.
- Algorithm Complexity: Developing quantum-ready trading algorithms demands expertise in both finance and quantum physics—a rare skill.
2. Data Privacy Risks
Quantum computers could eventually crack RSA encryption, threatening transactional security. The financial sector is investing in post-quantum cryptography to mitigate this risk.
3. Regulatory Uncertainty
Regulators struggle to keep pace with quantum innovations. Key questions include:
- How to audit quantum algorithms for fairness?
- Who is liable for errors in quantum-driven trades?
The Future of Quantum AI in Trading
1. Democratization of Quantum Tools
Cloud-based quantum platforms (e.g., Amazon Braket, Microsoft Azure Quantum) will let retail traders access quantum-powered analytics by 2030. Imagine a day trader using quantum simulations to optimize crypto trades from their laptop!
2. Hybrid Systems
Most firms will adopt hybrid quantum-classical systems, where quantum handles complex tasks (e.g., risk modeling), and classical AI manages execution.
3. Ethical and Regulatory Frameworks
Expect stricter guidelines for transparency, such as:
- Disclosing quantum algorithm use to investors.
- Third-party audits of quantum trading systems.
Conclusion: Embracing the Quantum Leap
Quantum AI isn’t a distant promise—it’s already reshaping trading floors, hedge funds, and retail platforms. From turbocharging predictive analytics to fortifying risk management, this technology offers a competitive edge that’s impossible to ignore.